Ricardo Baptista
Postdoctoral Scientist, Amazon Search
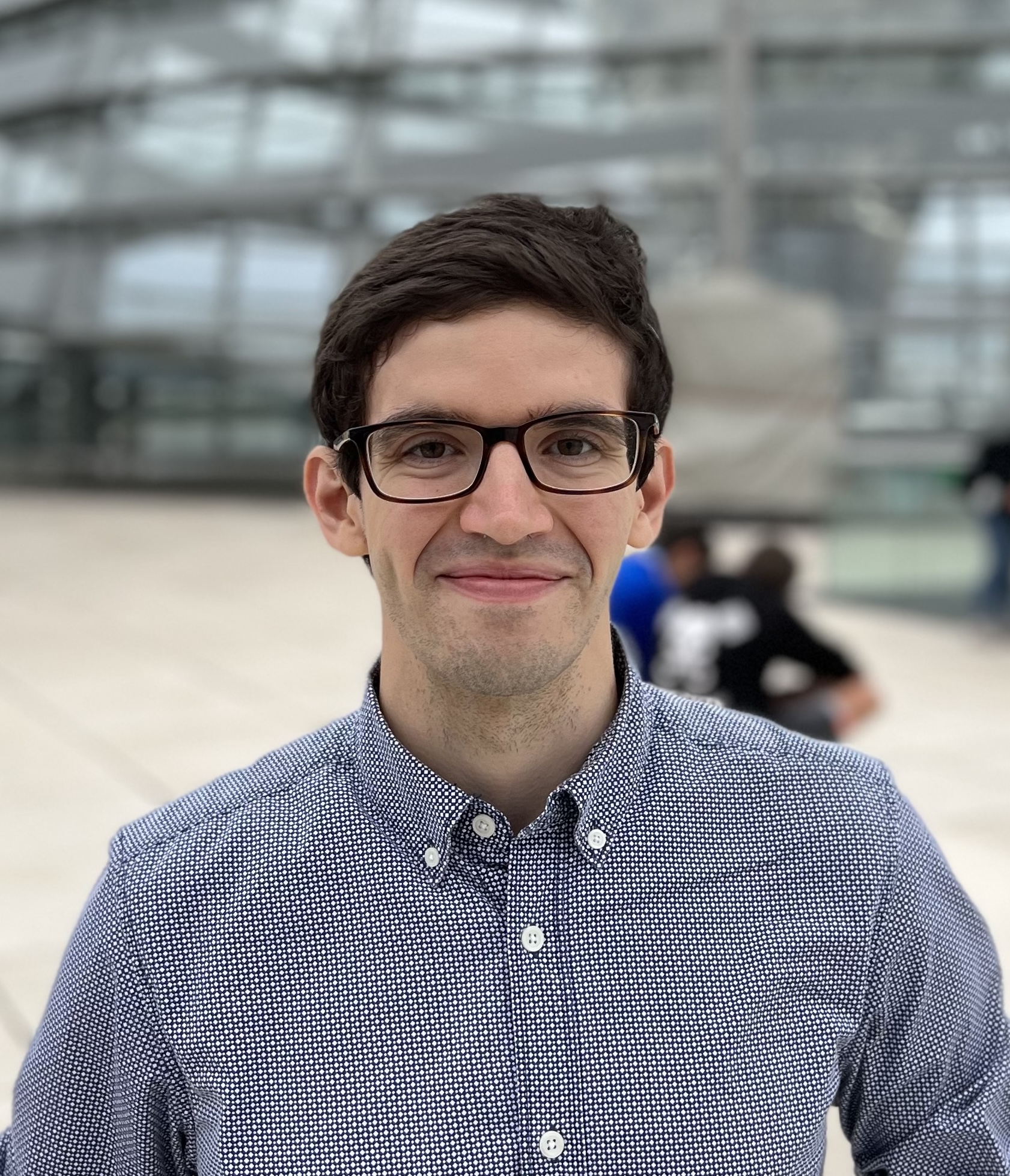
I am a Postdoctoral Scientist at Amazon and a Visitor at Caltech hosted by Andrew Stuart and Houman Owhadi. My research is on probabilistic modeling and inference for problems in science and engineering. Most recently, I have been developing and analyzing generative models based on computational measure transport.
In Fall 2025, I am excited to join the University of Toronto as an Assistant Professor in Statistical Sciences and Faculty Affiliate at the Vector Institute!
Bio: From 2022-2024 I was a von Kármán instructor at Caltech in Computing + Mathematical Sciences. I completed my PhD at MIT in Computational Science and Engineering where I was fortunate to be advised by Youssef Marzouk. A copy of my thesis can be found here. Before MIT, I received my BASc in Engineering Science from the University of Toronto.
Contact: rsb (at) caltech (dot) edu
Follow: Google Scholar arXiv GitHub LinkedIn
Announcements
Sep 2024 | Our paper on approximation theory for measure transport algorithms was accepted in AMS: Mathematics of Computations! |
---|---|
Jul 2024 | Gave a joint keynote talk at the CIRM-Marseille Digital Twins for Inverse Problems Workshop on Bayesian inference via dimension reduction. Thank you to all of the organizers! |
Nov 2023 | Gave the USNCCM Large-Scale TTA early-career colloquium on dimension reduction methods for probabilistic modeling. Thank you Shelly and Patrick for the invitation! |